5 key principles to keep ethical AI
- Ananya Kattyayan
- Aug 6, 2021
- 5 min read
Updated: Aug 30, 2021
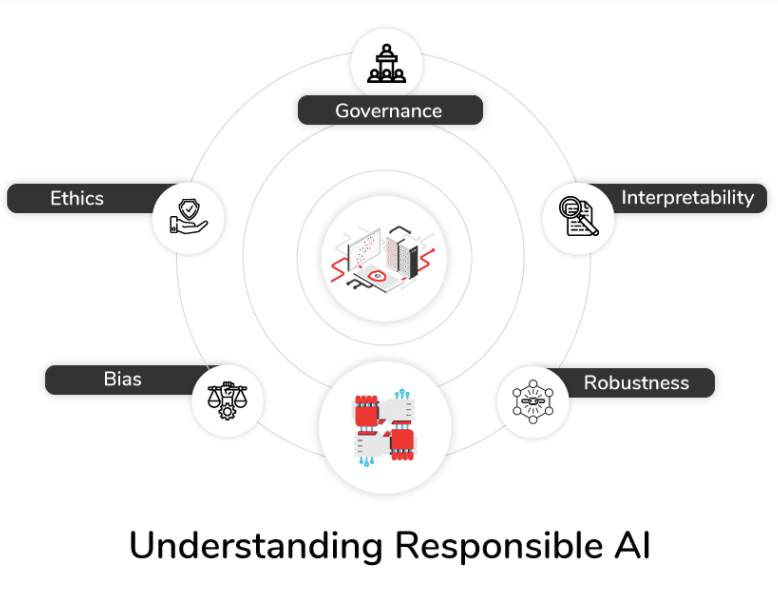
Responsible and Ethical AI isn’t a black & white concept. It is more like a million shades of grey. Call it a delicate balancing act. While you may regard a particular act to be responsible behavior, others may consider it rash. In the pursuit of one ethical objective, you might very well end up violating other moral principles. The odds are that you could be right, yet wrong, all at once. So, do you just give up? Do you just say, “Whatever, forget about ethics and responsibility”? A few years ago, you could have gotten away with that attitude. Not anymore. That approach can now expose your organization to massive reputational and legal risks. Some of the world’s largest corporations face intense scrutiny because of their AI systems’ perceived violations and biases. Your AI systems need to balance effectiveness with ethics. For instance, an e-commerce portal could make recommendations based only on maximizing profits without considering the value that a customer would derive from the offerings. That could be effective for the company, but customers may not view that to be ethical. So now how can we maintain ethical AI?
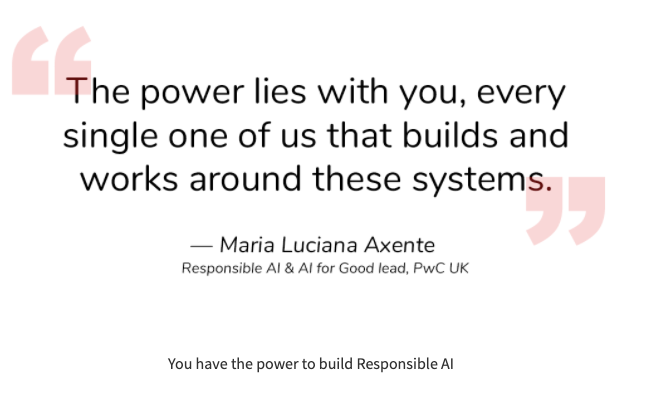
The principles of Responsible AI
1. Governance Who governs your AI systems? Do you have a team that makes sure these systems are used for good? Is there a committee that ensures that the data is validated? If you run a platform allowing others to use your AI systems, this is where the question of balance first comes into play. Can you check the information that your users are disseminating over your systems? Can you make sure that it’s validated and does not comprise unethical elements? While you could consider that to be a responsible practice, you’re running the risk of breaching your users’ privacy. It is the user’s responsibility to validate their data. However, we could receive reports about an account using our systems for unethical purposes. In such cases, we will flag this to the account owner. We will audit the bot and require the bot or account to be taken down if we feel it is necessary. 2. Interpretability Can you demonstrate how an AI model came to a specific conclusion or made a particular decision with absolute certainty? Is it possible to explain the exact reasoning behind every decision? Interpretability is critical when it comes to creating trustworthy AI systems. If you don’t know why a system is making a decision or offering a recommendation, you cannot blindly accept it. You will need to question that decision or suggestion. There are a few good tools that can precisely point out the reasoning behind a prediction every single time. But they are not as effective when it comes to complicated systems like Deep learning neural networks. Teams and organizations around the world are focusing their attention on this issue. They’re continuously researching and developing new tools and technologies that you can use to probe these predictions. Keep abreast of these developments and use these tools to the extent possible. At piMonk, we are always looking out for new tools to probe the decisions with greater precision. Currently, we run tests, take samples from them and use tools like ELI5, SHAP, and LIT to understand the features and reasoning behind the predictions. After that, our AI team looks into it to determine whether the features and logic used are appropriate for such an outcome. 3. Bias Does your AI model have biases based on gender, race, social class, or other social constructs? There’s a fair chance that it does.Every person has inherent biases. Your AI system may be exposed to the prejudices that the people who developed them hold. The systems could even pick up biases from the data that it’s trained on. Left unchecked, these biases can prove rather dangerous. In the world of finance, we’ve seen AI systems that offered higher credit limits to men than women. But it gets even scarier. There have been systems that allegedly encouraged healthcare workers to pay more attention to caucasian patients than others who needed attention more urgently. So, what can you do about it? First off, you need to evaluate and study the model to identify the biases to the extent possible. Educate yourself. Once you do that, you need to remove the training dataset’s bias and retrain the model. If you’re using a pre-trained model or it is not possible to retrain the model, you need to ensure that the applications you’re building cannot exploit the biases that the model holds. And if you can’t stop the application from exploiting the biases, you need to avoid that model like the plague. Whenever we add a new feature to piMonk, we try our best to ensure that the training data does not contain any biases. We have a strict review process to eliminate all evident biases from the data that the application uses. 4. Robustness Robustness is all about making sure that your AI systems consistently make the right decisions, avoid errors & biases and meet performance requirements. Ethics and values need to be at the core of our AI systems
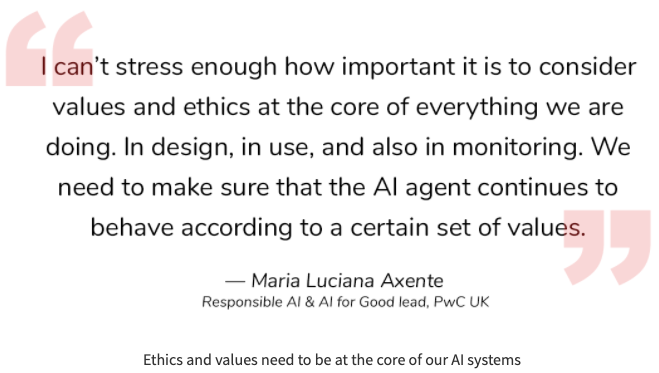
You need to keep updating and retraining your systems so that they don’t decline inaccuracy. Train your chatbot with FAQs
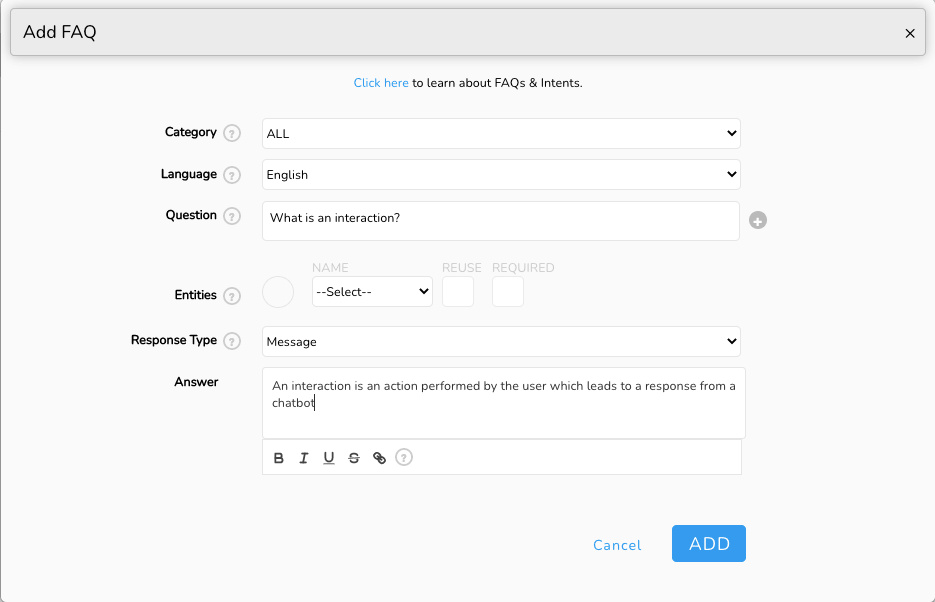
We then decided to simplify this process even further. By leveraging Cognitive Search, our DocuSense feature allows our customers to expedite bot training. All they need to do is upload a document (that doesn't even need to be in Q&A format). Their bot will then parse through the document and pull relevant information from it.
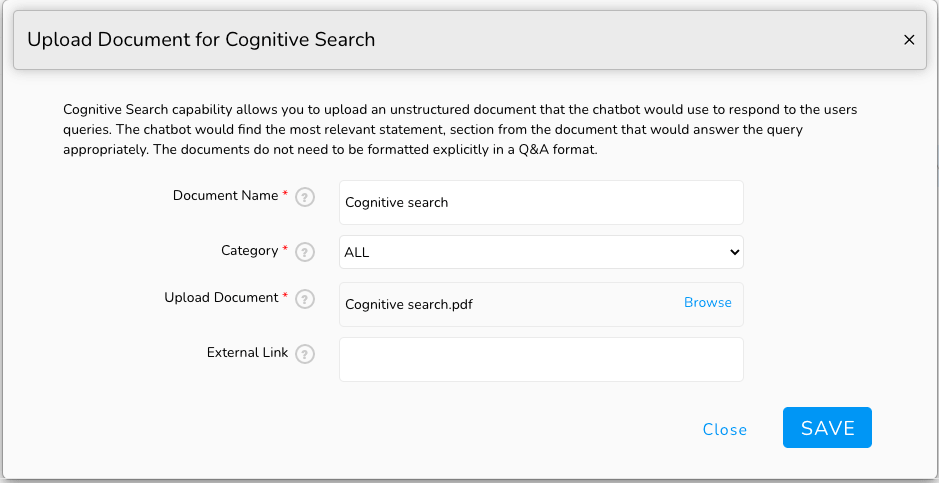
Train your chatbot with DocuSense 5. Ethics As noted above, Responsible AI is all about balancing effectiveness and ethical implications. Imagine if someone created an AI system that could map out which areas of forests could be wiped out with the most ease. The system could be powerful and effective. But its use can’t be considered ethical because it is a planet-unfriendly system.
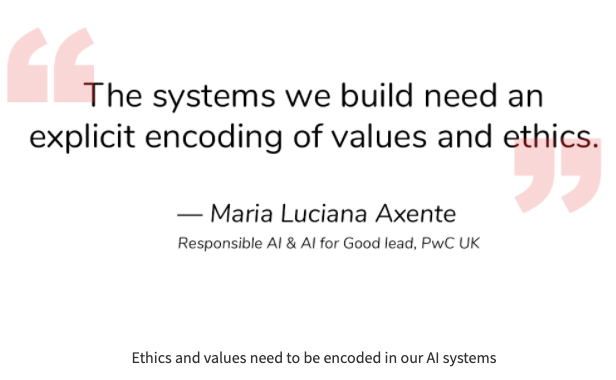
Ethics and values need to be encoded in our AI systems
An ethical AI system should do good for you and your users without harmful implications to others. If there are harmful implications, ask yourself, “how can they be minimized?”. Ethical AI is a journey. We are continuously educating our team about it and looking for new ways to make our AI systems as ethical as possible.
At piMonk, we take ethics seriously. We work with customers and partners across multiple industries to educate them and ensure that ethics are at the core of the solution being deployed.
That is something we believe that everyone who works in AI needs to do.
Getting educated about AI ethics Maria says that you need to be educated about AI ethics as soon as you start your computer science and AI journey. If you are going in for higher education, your curriculum needs to include ethical courses. If it doesn’t, you should demand them. Too many universities teach computer science but either don’t have an ethics course, or they’ve made it optional. Maria is a fan of Havard’s approach, where instead of creating a separate model on ethics in their computer science courses, the school has ethical considerations in every single module.
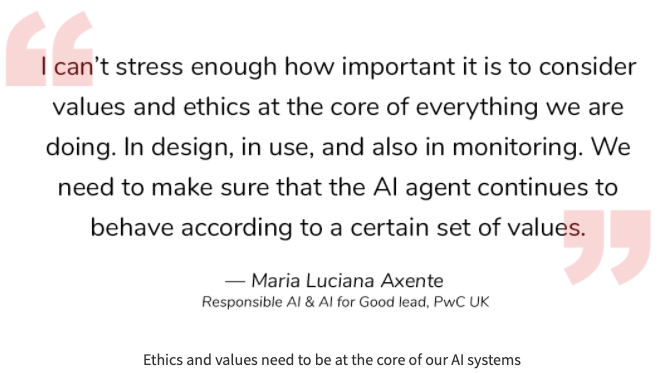
There are plenty of resources about Ethical AI, and you need to start exploring them as soon as you start learning about AI. According to Maria, right now, we are learning to fly while we are flying. It can get very challenging. We need to learn about ethical considerations as soon as we decide to get into the domain of artificial intelligence. So educate yourself as much as possible. Self-education is essential here.
Also, read our blog on Configuring Webhook in MS Teams
Comments